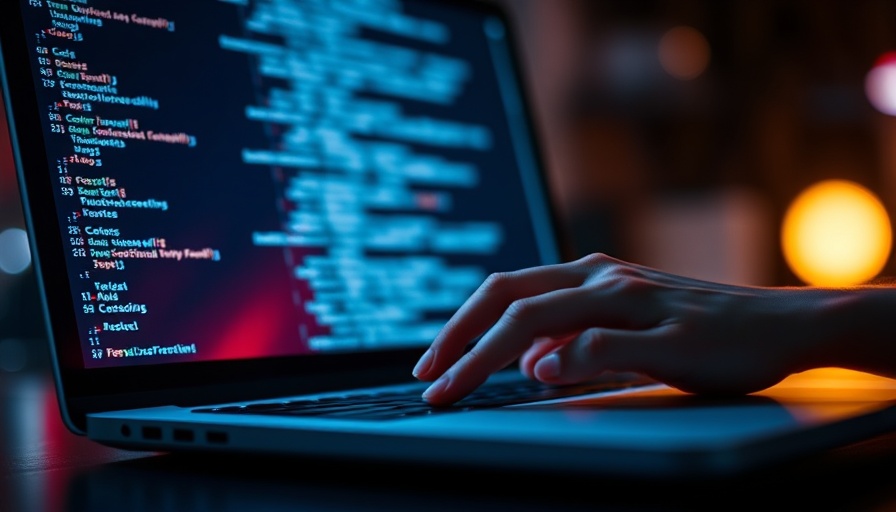
Understanding AI Model Performance Through Benchmarking
As businesses increasingly depend on artificial intelligence (AI) models, understanding how to measure and optimize their performance has become critical. Benchmarking AI models allows organizations to assess their capabilities, identify areas for improvement, and select the most suitable models for various tasks. This process involves setting clear evaluation criteria, conducting tests, and analyzing outcomes based on predefined metrics.
The Importance of Quality Benchmarks
High-quality benchmarks are foundational to effective AI evaluations. A recent study from Stanford University highlights that a good benchmark must meet several criteria throughout its life cycle—design, implementation, documentation, maintenance, and even eventual retirement. These criteria ensure that evaluations are not only consistent but also relevant to the tasks at hand.
According to the Stanford research, models are often optimally assessed at the design stage, while their performance may diminish during the implementation phase. Consequently, policymakers and developers must articulate and adhere to quality assurance practices when conducting or relying on AI evaluations.
Best Practices for LLM Benchmarking
With the advent of large language models (LLMs), benchmarking has become even more significant. Effective LLM benchmarking involves not just scoring models but also ensuring they are tested comprehensively across various tasks. Common techniques include few-shot and zero-shot testing, where models are assessed based on their ability to perform tasks with minimal instruction or without prior exposure.
Metrics like accuracy, recall, and the F1 score play vital roles in understanding model performance. These evaluations enable developers to tweak models, ensuring they are equipped to handle real-world scenarios adequately, thus enhancing user experience and satisfaction.
Strategies for Performance Tuning
Incorporating insights from analysts can significantly improve model performance. For instance, through continuous data collection and analysis, companies can refine their models to cater to specific market demands. This dynamic approach not only boosts efficiency but also ensures that the AI systems remain relevant and effective as technology evolves.
The Future of AI Evaluation
Looking ahead, organizations must stay abreast of emerging evaluation techniques and technologies. The rapid advancement of AI necessitates a proactive approach to benchmarking—one that evolves alongside technological breakthroughs. For instance, integrating AI models into broader digital transformation strategies can drive significant business growth and innovation in marketing tactics.
Practical Insights for Businesses
For business owners and marketers, understanding AI model benchmarking means better decision-making regarding technology adoption. Effective use of AI can enhance the customer experience, optimize marketing efforts, and boost operational efficiency. By leveraging benchmark assessments, companies can avoid the pitfalls of overfitting, ensuring their models perform well in real-world applications.
Moreover, knowledge gained from effective benchmarking can inform broader strategies in digital and content marketing. This encompasses everything from SEO updates to PPC advertising insights, as AI increasingly shapes the landscape of marketing decisions.
Insights Into the Evolving Digital Landscape
With the expansion of AI capabilities, marketers must consider the integration of AI performance insights into their overarching strategies. As they adopt AI tools for analytics and reporting, embracing continuous learning and adaptation will be essential for maintaining competitive advantages in a dynamically evolving market.
Conclusion: Next Steps in AI Benchmarking
To harness the full potential of AI technologies, businesses should invest in robust benchmarking practices and stay updated with advancements in AI performance metrics. By doing so, they'll not only improve their AI models but also significantly elevate their overall digital marketing strategies.
Write A Comment